
Unleashing the Power of Big Data to Revolutionize Risk Management

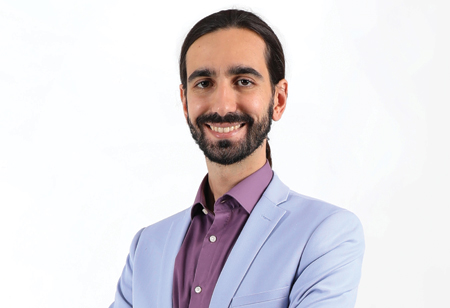
Kirill Odintsov, Head of Risk Underwriting and Data Analytics, Home Credit Indonesia, 0
Leveraging Big Data Analytics
Kirill emphasizes on big data analytics being crucial for risk management in financial institutions, focusing on non-traditional data like telco or app user behavior. This approach offers the needed understanding of customer risk which leads to precise predictions and increased customer approvals within risk appetite. Big data also aids in fraud reduction by utilizing third-party data, thus making it difficult for customers to provide inaccurate information. If we take an example, instead of directly asking for income information, financial institutions can access the data from credible external sources, enhancing reliability and streamlining the application process.
So if we look at markets like Indonesia, institutions have minimized mandatory fields, improving customer convenience. Furthermore, the impact of big data goes beyond risk management, positively affecting the customer journey and indirectly lowering risk by attracting higher-quality customers. Simplified application processes become tools for positive customer selection, optimizing risk management, increasing efficiency, and enhancing overall customer satisfaction.
In addition to aforementioned aspects, integrating big data into the scorecard further enhances risk management by statistically incorporating insights to predict customer default likelihood. This guides decision-making while influencing application approval, and supporting fair pricing. Moreover, leveraging big data refines risk predictions which enables precise underwriting decisions and accommodates individuals outside the traditional low-risk profile. Furthermore, managing data quality and integration in big data for risk management poses challenges. In my previous data science role, validating data from external partners, ensuring legal compliance, and obtaining consents were significant hurdles. Obtaining historical data from partners for back testing was a major challenge, hindering accurate evaluation.
Also, real-time data usage issues surfaced, impacting timely decision-making scenarios. Behavioral data from a client's app usage, though valuable, faced delays, reducing its impact. Ensuring legal compliance and obtaining consents added complexity, with partners' reluctance or inability to provide historical data posing a dilemma. And lastly, the demand for real-time decision-making in finance added urgency to data delivery. Some
external data sources provided valuable insights but with unacceptable delays, impacting timely, informed client-level decisions.
Enabling Thorough Risk Assessment
Big data plays a crucial role in risk management, particularly in leveraging standard application data for risk assessment. Behavioral and log data, capturing customer interactions, are essential for evaluating risk. Telecommunications companies collaborate, providing rich behavioral data like call duration and GPS coordinates. Privacy regulations are navigated through aggregated scores, ensuring individual customer information remains anonymous. Similar partnerships with e-commerce platforms and credit bureaus contribute to informed decision-making by financial institutions. Internally, innovative scores like the social circle score assess a client's risk based on their connections. Big data is also used to scrutinize customer behavior during the application process, identifying potential fraud or risky behavior and informing decisions on approval, pricing adjustments, and income verification for responsible lending.
Mitigating Challenges and Overcoming Hurdles
The talent and expertise gap in integrating big data into risk management poses a notable challenge for financial institutions. Addressing this challenge varies based on individual company policies and strategies. “In my experience, we prioritized nurturing internal talent when building the big data and data science team. Given the novelty of the field, finding experienced experts was challenging, and even those with experience often required specific training tailored to our company's needs. Our approach focused on creating comprehensive internal programs,” highlights Kirill Odintsov, Head of Risk Underwriting and Data Analytics at Home Credit Indonesia.
These programs included recruiting talented individuals, often from universities, and offering induction programs to teach them necessary skills. The goal was not only initial training but also ongoing development throughout their careers, ensuring a continuous pipeline of high-quality data and data science experts. Investing in the growth of internal talent enables financial institutions to overcome the shortage of experienced professionals in big data for risk management. This strategy aligns with the industry's evolving nature and the demand for specialized expertise in data-driven decision-making processes.
Professionals in big data and risk management must recognize its significance beyond a buzzword for staying relevant. Also to note, conducting a cost-benefit analysis before integrating big data is crucial, weighing its value against associated costs and ensuring only truly valuable data is utilized. Also, one should focus on data quality over model complexity, prioritizing good data with simpler models to avoid compromising insights. Moreover, the relevance and quality of data often supersede modeling complexity in the world of big data. This approach safeguards insights from being compromised by suboptimal data quality or irrelevant information,” concludes Kirill.
Enabling Thorough Risk Assessment
Big data plays a crucial role in risk management, particularly in leveraging standard application data for risk assessment. Behavioral and log data, capturing customer interactions, are essential for evaluating risk. Telecommunications companies collaborate, providing rich behavioral data like call duration and GPS coordinates. Privacy regulations are navigated through aggregated scores, ensuring individual customer information remains anonymous. Similar partnerships with e-commerce platforms and credit bureaus contribute to informed decision-making by financial institutions. Internally, innovative scores like the social circle score assess a client's risk based on their connections. Big data is also used to scrutinize customer behavior during the application process, identifying potential fraud or risky behavior and informing decisions on approval, pricing adjustments, and income verification for responsible lending.
Professionals in big data and risk management must recognize its significance beyond a buzzword for staying relevant.
Mitigating Challenges and Overcoming Hurdles
The talent and expertise gap in integrating big data into risk management poses a notable challenge for financial institutions. Addressing this challenge varies based on individual company policies and strategies. “In my experience, we prioritized nurturing internal talent when building the big data and data science team. Given the novelty of the field, finding experienced experts was challenging, and even those with experience often required specific training tailored to our company's needs. Our approach focused on creating comprehensive internal programs,” highlights Kirill Odintsov, Head of Risk Underwriting and Data Analytics at Home Credit Indonesia.
These programs included recruiting talented individuals, often from universities, and offering induction programs to teach them necessary skills. The goal was not only initial training but also ongoing development throughout their careers, ensuring a continuous pipeline of high-quality data and data science experts. Investing in the growth of internal talent enables financial institutions to overcome the shortage of experienced professionals in big data for risk management. This strategy aligns with the industry's evolving nature and the demand for specialized expertise in data-driven decision-making processes.
Professionals in big data and risk management must recognize its significance beyond a buzzword for staying relevant. Also to note, conducting a cost-benefit analysis before integrating big data is crucial, weighing its value against associated costs and ensuring only truly valuable data is utilized. Also, one should focus on data quality over model complexity, prioritizing good data with simpler models to avoid compromising insights. Moreover, the relevance and quality of data often supersede modeling complexity in the world of big data. This approach safeguards insights from being compromised by suboptimal data quality or irrelevant information,” concludes Kirill.